
Statistics major
Statistics is the science of collecting, managing, analyzing and interpreting data. Statistics is an essential tool in almost every field in undertaking research, product testing and development, quality control, and decision making.
Why major in statistics?
With the increase in data available to businesses, organizations and consumers, the need to make sense of that data has exploded. As a result, statistics is one of higher education’s fastest growing majors. Statistics requires excellent math skills, a sense of data structure and manipulation, and good problem-solving abilities. The combination of these areas enables statisticians to assist in research and discovery in almost every discipline. To researchers, statistics is a set of tools to help estimate effects and test hypotheses. To statisticians, the field is an exciting combination of theory, method and discovery to guide research and bring products to market faster.
There’s also plenty of statistician jobs to go around in the U.S. According to U.S. News and World Report, there are 10,500 jobs for statisticians. The median salary in 2022 was $98,920 and the unemployment rate was 0.3 percent.
10,500
jobs are available!
$98,920
The median salary in 2022.
What makes our program unique?
UD will allow you to apply statistical techniques to real data and real problems. So you will be highly sought after when it’s time to enter the job market. Our students build a “statistical imagination” in order to address a range of problems in diverse fields. At UD, you will build a firm foundation in statistical theory, complemented by courses in applied statistics and data management using SAS, R, JMP. Our courses apply to problem solving in areas like economics, biology, business or the environment. Couple this major with a minor in resource economics, and you’ll land an impactful, high-paying job upon graduation!
In addition to the required core courses of the major, you’ll choose an “area of application” for further in-depth study. Starting your sophomore year, you’ll dive into a subject area of interest to you and earn the equivalent of a minor in that discipline. Examples include:
Advertising,
Bioinformatics,
Business administration,
Business analytics,
Cognitive science,
Computational biology,
Computer science,
Economics,
- Game studies,
- International business studies,
- Management information systems,
- Organizational and community leadership, and
- Resource economics.

Program goals
Upon completion of the Statistics (B.S.) major, students can expect to:
Demonstrate understanding of mathematical concepts and techniques used in statistical theory and applications.
Apply analytical methods of data exploration, graphical methods, descriptive methods, and introductory statistical inference.
Apply statistical modeling, statistical analyses, and advanced statistical inference to diverse business and science applications.
Demonstrate proficiency in various modern programming languages and statistical software to manipulate data and facilitate statistical computing.
Exercise well-developed critical thinking and oral and written communication skills in order to provide effective analysis of real-world questions.
Demonstrate responsible and ethical professional conduct that respects diverse perspectives and promotes inclusivity.
Will a statistics major prepare me to be a data scientist?
Absolutely. A major in statistics at UD will prepare you for careers or further study in data science, as well as many other fields. Statistics is the basic mathematical science behind data science. It is, in short, the science of data. Statistics refers to every aspect of how we handle and use data such as collecting data; classifying, summarizing, and organizing data; analysis of data using summary measures and graphs; making inferences from a sample to a population; and interpretation of the results. Statistics is both a field of study and a set of tools used by many disciplines, such as business, economics, health sciences, and the social sciences. We provide you the tools to be a data scientist by providing you the technical background in mathematics, statistics, and computer science that you need. But only in Statistics will you get the depth of knowledge in advanced statistical techniques that makes you a Statistician.
For more information, visit the UD Online Catalog.
Career Outcomes
Career paths
Risk Assessment Analyst
Market Researcher
Pricing Analyst
Federal Statistics Statistician
Credit Analyst
Sports Statistician
Product Development Analyst
Graduate school paths
Data Science
Business Analytics
Biostatistics
Economics
Resource Economics
Education Statistics
The job market is so hungry for statisticians
Courses and planning
Course highlights
Students use data from a variety of disciplines to explore topics in statistical data analysis, estimation, and inference. Instructors cover graphical displays; measures of position, central tendency, and variability; basic probability rules; discrete probability distributions; binomial distribution; normal and standard normal probability distributions; sampling distributions; the t distribution; confidence intervals and hypothesis tests for one mean or proportion; confidence intervals and hypothesis tests for two means or proportions; correlation and simple linear regression.
Students gain an overview of inferential methods to analyze regression models and linear models for experimental designs.
Students will conduct statistical analysis of data in an individual project they’ve chosen and had approved by their instructor. The student will propose their own project, collect data, manage data, conduct statistical analysis, write a formal paper describing the process and results, and present their research project to the class.
Instructors focus on calculus-based probability theory as typically applied to statistical analyses. This course is part of a two-semester sequence (STAT470 and STAT471) that serves as the theoretical foundation for statistics majors.
Students will learn about statistical programming languages, which may include SAS and R.
A 4-Year Plan
This sample shows just one possible pathway to earning a bachelor of science degree in Statistics in four years. This plan does not replace the advice of your advisor.
Related student organizations
Apply and Learn More
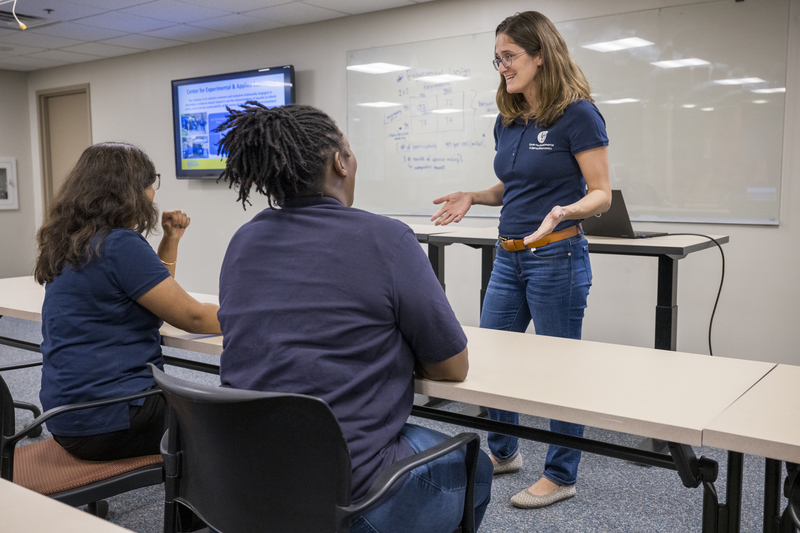
APPLY NOW
Associate Director, CANR Undergraduate Recruitment