
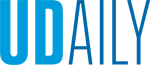
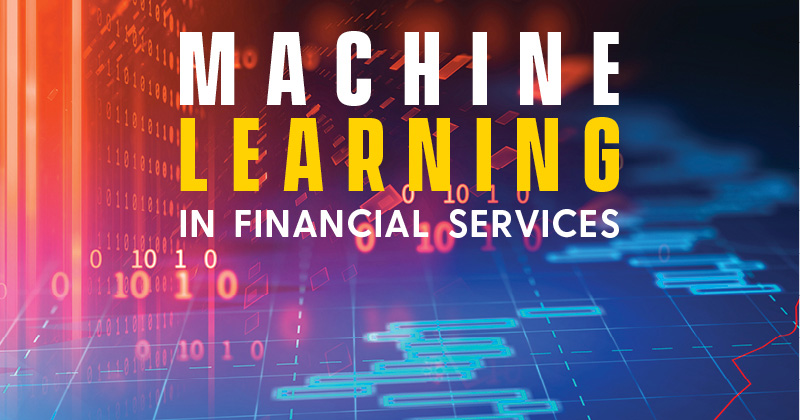
Machine learning in financial services
Photos by Kathy F. Atkinson | Image by Getty Services November 27, 2019
UD conference connects industry and academia to consider the possibilities
The financial services industry is using machine learning to revolutionize its processes and rapidly improve financial outcomes, and its potential seems limitless. That’s why the University of Delaware’s Institute for Financial Services Analytics (IFSA) brought together leading minds in industry and academia for its 2019 conference “Machine Learning in Financial Services,” hoping to create a dialogue and in which both can learn from each other and explore this potential.
“Machine learning can solve a lot of problems, but there are also challenges,” said Bintong Chen, UD professor of operations management, IFSA director and faculty director for the financial services analytics (FSAN) doctoral program. “This theme was chosen, first, because this technique is getting popular and there is an abundance of data, fast computing and awareness. Second, it is closely related to research that our faculty and students are conducting at IFSA.”

Machine learning is an area within data science where statistical models are used to make predictions and develop solutions. These unique models are able to take the inputted data and automatically work to improve outcomes. Data scientists and industry experts are able to train machine learning models to function in real-world scenarios to help find important solutions.
According to Chen, although machine learning has been widely used in the financial services industry, within higher education he wasn’t aware of a conference that would delve deeper into research around machine learning. IFSA is a top research center at UD whose faculty and students in the first of its kind FSAN doctoral program tackle many different data challenges of the financial services industry. The team at IFSA decided to leverage this unique position within financial services to fill this gap.
By connecting industry with academia, the goal of this conference was to start a conversation around machine learning as a tool that experts can study and use to achieve real-world industry goals like decreasing operational costs, increasing productivity and more. Conference attendees included professionals from JPMorgan Chase & Co. (JPMC), Bank of America, Citibank, Cyber 2020, Sallie Mae, Vel Micro and other financial service institutions as well as those from UD, Delaware State University and other academic centers.
Machine Learning Today
For conference presenters, Prashant Dhingra, head of development for machine learning at JPMC, and Sanchit Arora, head of machine learning and data science at Possible Finance, machine learning is part of their day-to-day operations.
Dhingra shared lessons that he had learned from working on hundreds of machine learning projects for JPMC. He focused on how firms can overcome challenges that may arise from integrating machine learning into processes within an enterprise. Dhingra also discussed challenges that emerge at every stage, from experimentation through operationalization, and how his team has been able to adjust to or overcome them.
Arora described how Possible Finance uses machine learning in its technology with the goal of helping Americans improve their financial well-being by providing affordable short term loans that build credit. He discussed the potential for machine learning to help solve complex problems and automate processes while remaining fairer and more transparent than other methods.
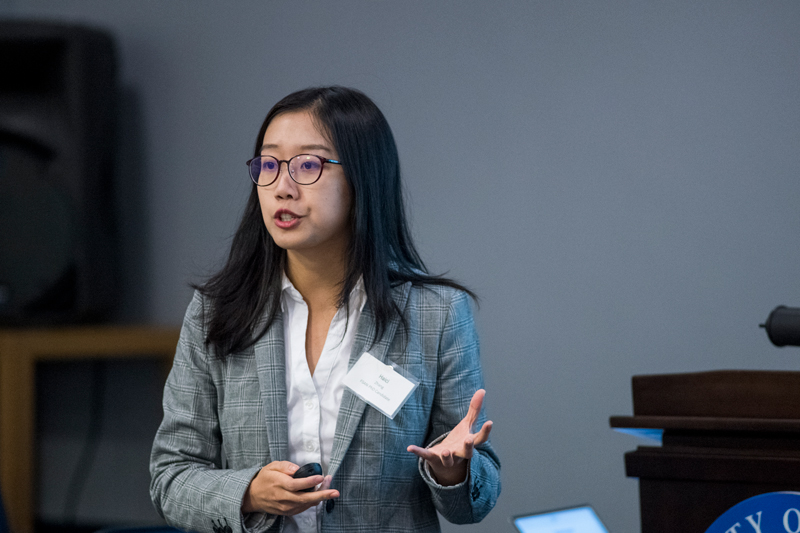
One area that machine learning can have a practical application on consumers and industry is generating credit scores. Two conference presenters focused their presentation on this innovative industry use of machine learning.
Stefania Albanesi, a professor of economics at the University of Pittsburgh, presented her research on the potential value that machine learning may have for predicting consumer default on loans. Using a deep learning approach, Albanesi and her research partner developed a model to predict consumer default. This model has consistently outperformed standard credit scoring models. As it continues, this research may have practical implications for both the financial services industry and consumers.
Where Albanesi, from an academic research perspective, presented a potential model for replacing traditional means of credit reporting, Sarah Davies, head of credit risk and analytics for the cross-border credit reporting agency Nova Credit, explained the value of machine learning within those existing credit risk score development processes. She showed how the use of machine learning optimized her company’s performance at predicting consumer credit risk. Davies outlined the various challenges to and opportunities for machine learning in this industry based on scenarios she has experienced in her work.
The Future of Machine Learning
Machine learning, however, is still a largely untapped resource. Students in the FSAN doctoral program at UD’s Alfred Lerner College of Business and Economics discussed their current research in machine learning through networking sessions and select presentations. These sessions allowed the conference attendees to learn about possible future applications of these tools to their industry while giving the students a chance to network and receive expert feedback on their work.
Paul Laux, professor of finance, introduced the John Cavazos Student Research Presentation Session. This session was named for John Cavazos Jr., an associate professor in UD’s Department of Computer and Information Sciences and Department of Electrical and Computer Engineering and a JPMC faculty fellow for IFSA, who died in 2018.
“John was in the group that had the idea for the FSAN program,” Laux said. “One of the really important things about John is that even in his last days he was working closely with his students. We decided to name this session, which he would have loved, in his honor today.”
The first student presenter, Jared Sharpe, discussed his research on modeling within the real estate market. Sharpe used agent-based modeling to predict how real estate agents can affect the average sale price, number of homes sold and buyer and seller satisfaction. Using real-world data to create a simulated model, Sharpe’s model showed how real estate agents can be market influencers.
Haici Zhang, the second student presenter, shared the details of her research about the interbank market. The interbank lending market is essentially where banks extend loans to one another, which was a major factor in the financial crisis in 2008. Through her research, Zhang created a Bayesian dynamic interbank network model that has a higher prediction power for bank linkage than other models to predict economic uncertainty index.
The final student speaker, Xiaohang Zhao, took a deep learning approach to industry classification systems (ICSs). According to Zhao, ICSs identify economically related firms as peer firms and are essential for providing peer-firm information. Through his research, Zhao developed a novel ICS which, through empirical evaluations, demonstrates superior results in providing a more scalable and effective approach to determining peer firms than traditional models. This research won the best paper award at the 2019 INFORMS Workshop on Data Science, the premier academic conference for data science and business analytics.
This conference opened lines of communication that will continue through the work of IFSA and the financial services industry throughout Delaware. There is still more to be discussed, discovered and shared between industry and academia in the financial services space in order to continue to effectively integrate machine learning into the industry processes. IFSA is currently recruiting those interested in becoming the next pioneers in machine learning for its fourth FSAN doctoral cohort.
Contact Us
Have a UDaily story idea?
Contact us at ocm@udel.edu
Members of the press
Contact us at 302-831-NEWS or visit the Media Relations website